Assumptions Of Chi Square

The Chi-square test, a powerful statistical tool, is widely employed in various fields to analyze categorical data and make inferences about populations. Understanding the assumptions behind this test is crucial for ensuring accurate and reliable results. In this comprehensive article, we delve into the key assumptions of the Chi-square test, exploring their implications and providing real-world examples to enhance our understanding.
The Assumptions of Chi-square Test

The Chi-square test, often denoted as χ², operates under several fundamental assumptions that are essential for its validity and applicability. These assumptions serve as the foundation for interpreting the test's outcomes accurately.
Independence of Observations
The first assumption is that the observations or data points being analyzed are independent of each other. In other words, the outcome of one observation should not influence or be dependent on the outcome of another. This assumption is critical as it ensures that each observation contributes unique information to the analysis.
Consider a study investigating the relationship between smoking habits and lung cancer. If the same individuals are repeatedly surveyed over time, their responses may become correlated, violating the independence assumption. To address this, researchers often employ cross-sectional studies, where data is collected from a diverse sample at a single point in time.
Random Sampling
The Chi-square test assumes that the data has been collected through random sampling. This means that each member of the population has an equal chance of being selected for the sample. Random sampling helps ensure that the sample accurately represents the entire population, reducing the potential for bias.
For instance, imagine conducting a survey on student preferences for different lunch options in a school. To ensure random sampling, the researchers might assign each student a unique number and use a random number generator to select participants. This approach minimizes the influence of personal preferences or social dynamics on the sample selection.
Expected Cell Counts
The Chi-square test is sensitive to the distribution of data within the expected cell counts. Expected cell counts refer to the theoretical values that would be expected if there were no relationship between the variables being analyzed. These counts are calculated based on the observed data and the assumed independence of the variables.
In the context of a study examining the relationship between gender and preferred sports, the expected cell counts represent the number of males and females who would participate in each sport if gender had no influence on sport preference. Deviations from these expected counts can indicate a relationship between the variables.
Gender | Soccer | Basketball | Swimming |
---|---|---|---|
Male | 50 | 40 | 30 |
Female | 40 | 50 | 40 |
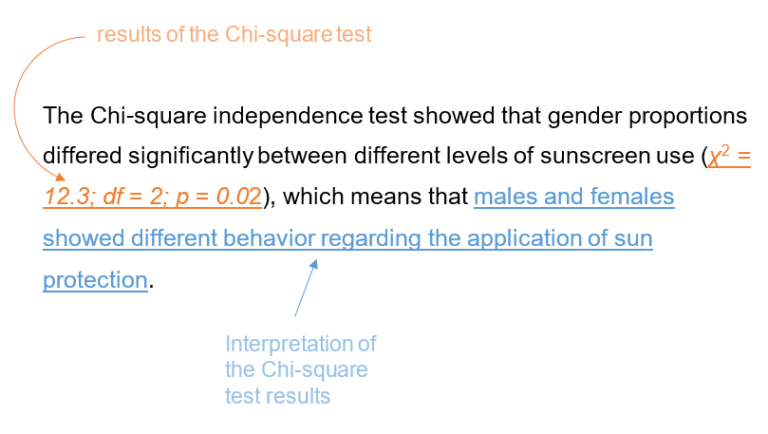
Large Sample Size
The Chi-square test is most effective when applied to large sample sizes. While there is no strict threshold for a "large" sample, generally, a sample size of at least 50 is considered preferable. Smaller sample sizes may lead to unreliable results, especially when dealing with expected cell counts that are too small.
Imagine a study comparing the effectiveness of two teaching methods in a small classroom of 10 students. With such a limited sample, it becomes challenging to draw meaningful conclusions, as the results may be heavily influenced by individual variations or random fluctuations.
Categorical Data
The Chi-square test is specifically designed for analyzing categorical data, which consists of variables that can be classified into discrete categories or groups. These categories should be mutually exclusive, meaning that each observation can only belong to one category.
For example, in a study assessing the impact of different marketing strategies on customer engagement, the categorical data might include variables such as customer age groups (e.g., 18-25, 26-35, 36+), gender, and response to the marketing campaign (positive, neutral, negative).
Cell Count Requirements
To ensure the validity of the Chi-square test, certain cell count requirements must be met. Specifically, the test assumes that no cell count should be zero, and most cell counts should be at least 5. Having zero cell counts or too many small cell counts can lead to unreliable results and potentially inflate the significance of the test.
Consider a study examining the relationship between socioeconomic status and educational attainment. If the sample includes a significant number of individuals from lower socioeconomic backgrounds, but none from higher-income families, this would result in a zero cell count, violating the assumption.
Assumption of Homogeneity
The Chi-square test assumes that the observations within each category are homogeneous, meaning they exhibit similar characteristics or behaviors. This assumption is crucial when comparing multiple categories or groups.
For instance, in a study comparing the effectiveness of different study techniques among students, the assumption of homogeneity implies that the students within each study technique group are similar in terms of their academic abilities, motivation, and other relevant factors.
Implications and Considerations
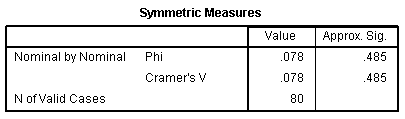
Understanding and addressing the assumptions of the Chi-square test are essential for conducting rigorous statistical analyses. Violating these assumptions can lead to misleading results and incorrect conclusions. Researchers must carefully consider the nature of their data and study design to ensure that the assumptions are met or develop alternative approaches when necessary.
Additionally, while the Chi-square test is a valuable tool for categorical data analysis, it is not without its limitations. For more complex data or when dealing with continuous variables, alternative statistical tests such as analysis of variance (ANOVA) or regression analysis may be more appropriate. Researchers should choose the most suitable test based on the nature of their data and research questions.
In conclusion, the assumptions of the Chi-square test provide a framework for ensuring the validity and reliability of statistical inferences. By carefully considering these assumptions and employing appropriate data collection and analysis techniques, researchers can confidently draw meaningful insights from their studies, contributing to the advancement of knowledge in their respective fields.
What happens if the independence assumption is violated?
+
If the independence assumption is violated, it can lead to biased results and incorrect conclusions. It’s crucial to ensure that observations are independent to maintain the validity of the Chi-square test.
How can I ensure random sampling in my study?
+
Random sampling can be achieved through various methods, such as random number generation, random selection from a population list, or using probability-based sampling techniques. These approaches help ensure that each member of the population has an equal chance of being selected.
What if my sample size is smaller than recommended for the Chi-square test?
+
While the Chi-square test is most effective with larger sample sizes, smaller samples can still provide valuable insights. However, it’s important to exercise caution and consider the potential limitations of the test with smaller samples. In such cases, alternative statistical methods may be more suitable.